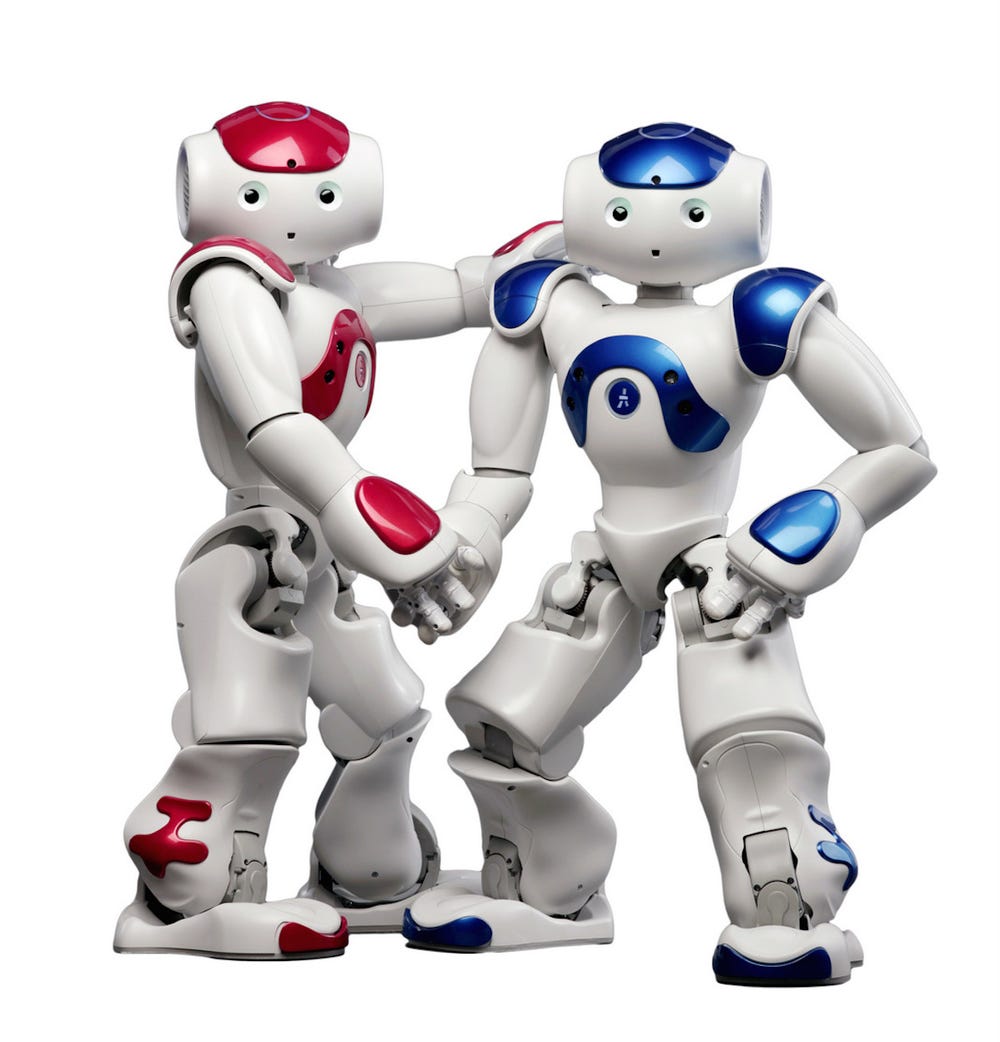
Transfer learning is a powerful tool to help companies adapt to the changes in their workforce. The process involves using machine learning algorithms to identify subjects in new contexts. These algorithms can be retained in their entirety, making it less difficult to recreate them. Here are some techniques for applying transfer learning to business:
Techniques
Transfer learning is an approach to computer science that allows models of machine learning to be trained by using the same data set or similar. For example, natural language processing uses a model capable of recognizing English speech in order to recognize German speech. A model that was trained to drive autonomous cars can be used in order to identify different types of objects. Even if the target language is different, transfer learning can help improve the performance of machine learning algorithms.
"Deep transfer learning" is a very common technique. This method can be used to teach similar tasks to different datasets. The technique allows neural network to learn quickly and easily using previous experiences, thus reducing training time. As a result, transfer learning algorithms are much more accurate and less resource-intensive than training new models from scratch. Many researchers are discovering the many benefits of transfer learning as this process has grown in popularity.
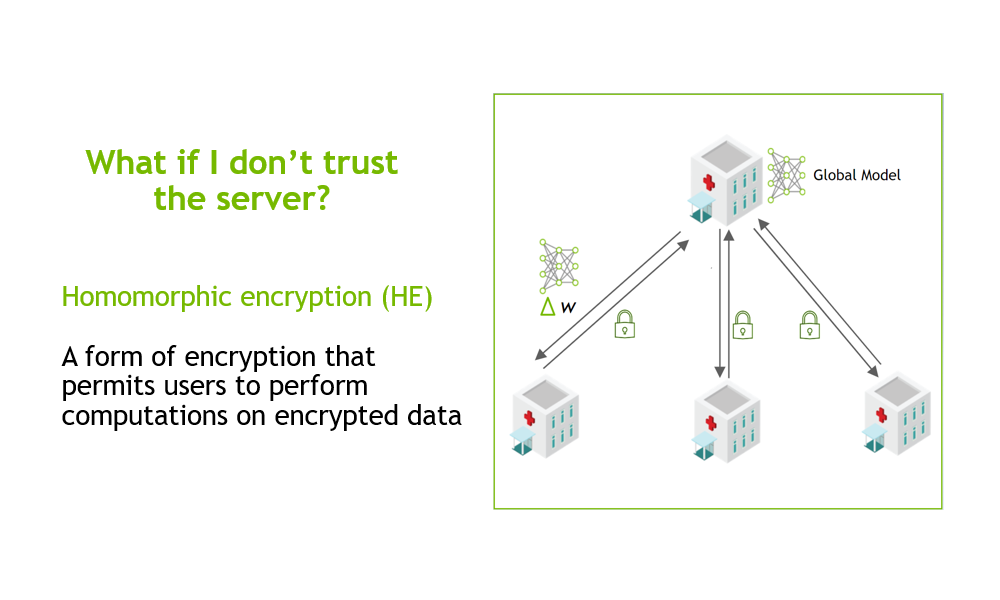
Tradeoffs
Transfer learning refers to a cognitive process that a learner uses to combine knowledge from one domain and another. Transfer learning is a combination of observation in the target domain as well as knowledge from the source domain. These same strategies can be used to build the model. The method comes with some tradeoffs. In this article, we will discuss the tradeoffs that can be made with different learning environments. This article will help you evaluate the effectiveness of different transfer learning strategies.
One of the main drawbacks of transfer learning is that it degrades the performance of the model. Negative transfer occurs when a model is trained with large amounts of data but cannot perform well in its target domain. The danger of transfer learning is overfitting. This can lead to overfitting in machine learning, which is when the model learns more from the training data than it should. Therefore, transfer learning is not always the best approach for natural language processing.
Indications of effectiveness
Transfer learning has many advantages. It is a great way for neural networks to be built and trained in many areas. It can also be applied to empirical Software Engineering, which is difficult because large, labeled datasets don't exist. Practitioners can also use it to construct deep architectures with minimal customization. Indications of effectiveness of transfer learning vary, but they all point to a successful outcome. These are just three examples.
Comparison of their performance across different datasets was used to evaluate the performance of the models. The results were varied in terms of success. Transfer is more effective than unsupervised training when there are large data differences. Both methods are best suited for large datasets. Transfer learning is measured by several metrics such as accuracy, specificity and sensitivity. This article will focus on the key findings of supervised and transfer learning.
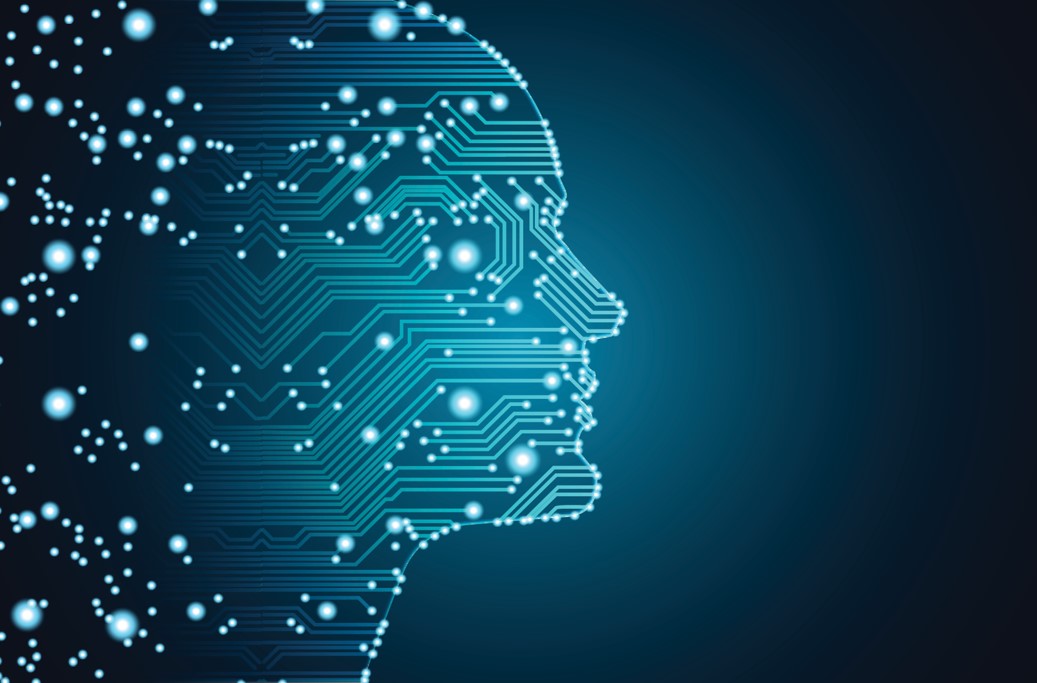
Applications
Transfer learning allows you to transfer a model from one task into another. A model that was trained to detect car dings may be used to detect buses and motorcycles. This knowledge transfer works well in ML tasks when the models have similar physical property. This knowledge transfer can be used to enhance the performance of machine learning systems. What applications can transfer learning have? Let's discuss some of them.
NLP is one popular application for transfer learning. Its key advantage is the ability to leverage the knowledge of existing AI models. This allows the system to learn how to optimize certain outcomes of textual analysis. The most common problem in sequence labeling involves taking text as input and predicting a sequence that contains named entities. These entities can easily be classified and recognized by word-level representations. This process can be dramatically shortened using transfer learning.
FAQ
Which industries use AI the most?
Automotive is one of the first to adopt AI. BMW AG employs AI to diagnose problems with cars, Ford Motor Company uses AI develop self-driving automobiles, and General Motors utilizes AI to power autonomous vehicles.
Other AI industries include banking, insurance, healthcare, retail, manufacturing, telecommunications, transportation, and utilities.
Is AI good or bad?
AI is both positive and negative. AI allows us do more things in a shorter time than ever before. Programming programs that can perform word processing and spreadsheets is now much easier than ever. Instead, our computers can do these tasks for us.
People fear that AI may replace humans. Many believe that robots will eventually become smarter than their creators. This could lead to robots taking over jobs.
Are there any risks associated with AI?
Of course. There will always be. Some experts believe that AI poses significant threats to society as a whole. Others argue that AI is not only beneficial but also necessary to improve the quality of life.
AI's greatest threat is its potential for misuse. The potential for AI to become too powerful could result in dangerous outcomes. This includes autonomous weapons and robot rulers.
AI could also take over jobs. Many people are concerned that robots will replace human workers. Others believe that artificial intelligence may allow workers to concentrate on other aspects of the job.
Some economists even predict that automation will lead to higher productivity and lower unemployment.
Which countries are leading the AI market today and why?
China has the largest global Artificial Intelligence Market with more that $2 billion in revenue. China's AI industry is led Baidu, Alibaba Group Holding Ltd. Tencent Holdings Ltd. Huawei Technologies Co. Ltd., Xiaomi Technology Inc.
China's government is investing heavily in AI research and development. The Chinese government has created several research centers devoted to improving AI capabilities. These include the National Laboratory of Pattern Recognition and State Key Lab of Virtual Reality Technology and Systems.
China is home to many of the biggest companies around the globe, such as Baidu, Tencent, Tencent, Baidu, and Xiaomi. These companies are all actively developing their own AI solutions.
India is another country making progress in the field of AI and related technologies. India's government is currently focusing its efforts on developing a robust AI ecosystem.
What is AI good for?
There are two main uses for AI:
* Prediction – AI systems can make predictions about future events. AI systems can also be used by self-driving vehicles to detect traffic lights and make sure they stop at red ones.
* Decision making – AI systems can make decisions on our behalf. As an example, your smartphone can recognize faces to suggest friends or make calls.
Statistics
- More than 70 percent of users claim they book trips on their phones, review travel tips, and research local landmarks and restaurants. (builtin.com)
- By using BrainBox AI, commercial buildings can reduce total energy costs by 25% and improves occupant comfort by 60%. (analyticsinsight.net)
- The company's AI team trained an image recognition model to 85 percent accuracy using billions of public Instagram photos tagged with hashtags. (builtin.com)
- A 2021 Pew Research survey revealed that 37 percent of respondents who are more concerned than excited about AI had concerns including job loss, privacy, and AI's potential to “surpass human skills.” (builtin.com)
- While all of it is still what seems like a far way off, the future of this technology presents a Catch-22, able to solve the world's problems and likely to power all the A.I. systems on earth, but also incredibly dangerous in the wrong hands. (forbes.com)
External Links
How To
How do I start using AI?
A way to make artificial intelligence work is to create an algorithm that learns through its mistakes. This learning can be used to improve future decisions.
For example, if you're writing a text message, you could add a feature where the system suggests words to complete a sentence. It would learn from past messages and suggest similar phrases for you to choose from.
However, it is necessary to train the system to understand what you are trying to communicate.
Chatbots can be created to answer your questions. If you ask the bot, "What hour does my flight depart?" The bot will answer, "The next one leaves at 8:30 am."
If you want to know how to get started with machine learning, take a look at our guide.