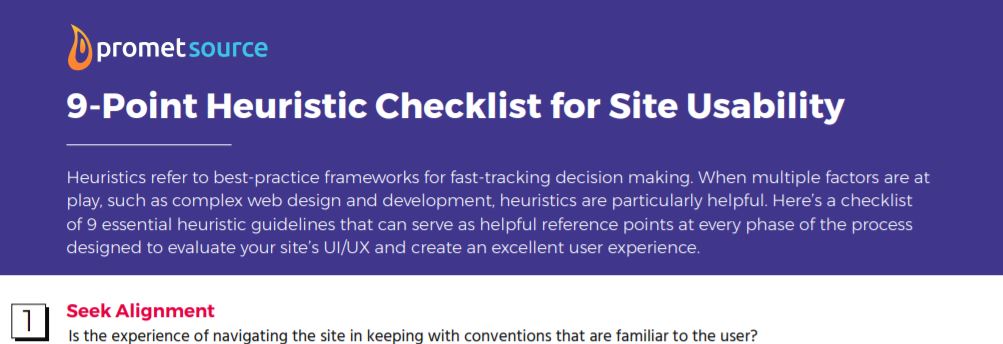
Because of their many benefits, machine learning video games are growing in popularity. For example, the recently released game "Simon's Clash" uses AI technology to recognize lost players and retry them. Researchers were not able to prove this technique was as efficient as they had hoped. Low performance could be due either to the complexity or ambiguity surrounding the word "lost".
Artificial Neural Networks
Artificial Neural Networks (ANNs) are an example of how deep-learning algorithms can improve esports game AI. The videogame industry is rich in data, which can be used to create machine learning algorithms. DeepMind has, for instance, used video games to create AI systems that are capable of defeating e-sports pros. Researchers can use machine learning algorithms to improve their performance in video games.
The learning process is very different for curiosity-driven and extrinsically-motivated neural networks. Curiosity driven neural networks learn from analyzing the actions of the player and the outcomes. They can predict the future and minimize predictions errors. In this way, they are more efficient than extrinsically-motivated neural networks. AI used for video games is thus advancing in many aspects.
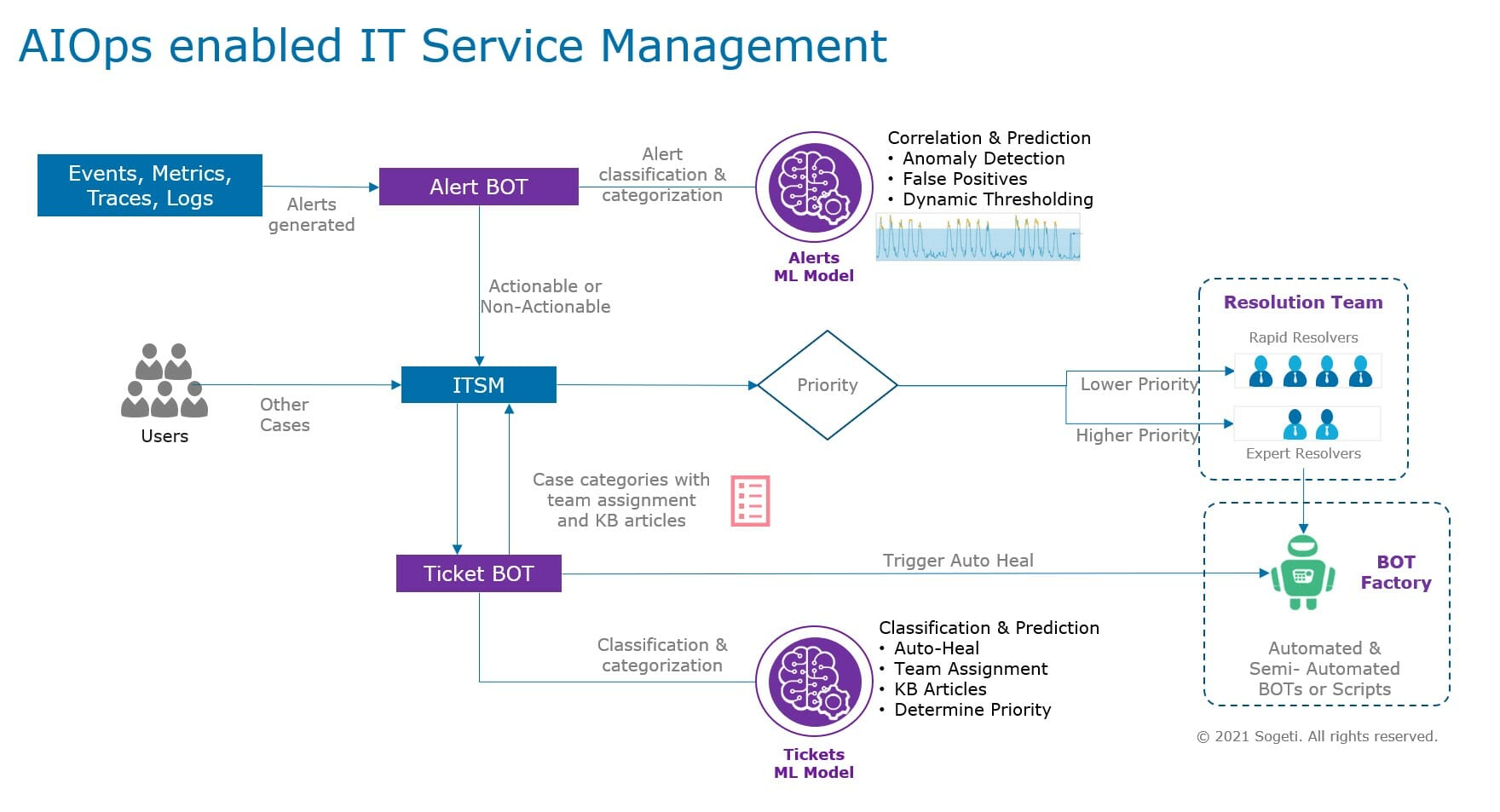
Genetic algorithms
Genetic algorithms were developed as a result of the development of artificial intelligence. These algorithms take a number of steps to solve a problem. They include mutation and selection. These algorithms are applicable in many areas, such as economics, multimodal optimizing, aircraft design, DNA analysis, and even economics. This article will provide a general overview of how these algorithms work and some of their limitations. Let's look at the role of genetic algorithms for machine learning video games.
Fitness function is an important parameter. The more fitness you have, the better your solution. The algorithm will also need to calculate the distance between two solutions. This is done using the current locations of objects. This allows the user to create a fitness function. Important to know that fitness values are used for evaluating the performance of the solution. A fitness function will allow the user to make an informed decision on which solution is the best.
N-grams
Researchers are using n-grams more often to train game algorithms. Unlike standard machine learning techniques, which rely on large amounts of data, n-gram models are based on a single-dimensional input - a string. Researchers first need to convert levels into strings in order to train ngram models. These strings are then made into vertical slices. Each piece repeats several times. The model calculates a conditional probability for each character.
For text data, the concept of ngrams was created. Grayscale can be defined as any range of values between zero and 255. This is equivalent to a dictionary that contains 256 words. In a text, there can be as many as 2256 possible ngrams. High-dimensional data on the other side is susceptible to information redundancy and noise as well as dimensional disasters. N-grams serve as prefix searching and for the implementation of a "search-as_you-type" system.
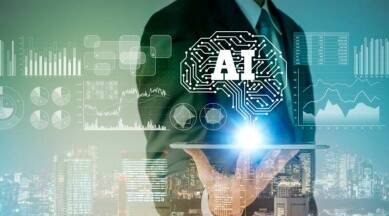
Training data
It's a difficult task to develop AI techniques for videogames. You need to have a lot of training data. Although game developers have the option to use their own data for models of player behavior and machine learning techniques can be used to learn from examples of video games, they are not able to do so without extensive training data. Game developers can analyze game data to create systems that can learn from a variety of scenarios and play games with varying difficulty. Developers can also incorporate machine learning techniques in the design of their games.
An AI model can be created in the same way as a program to play chess. Machine learning is however at a higher level. Machine learning techniques can be trained from synthetic data rather than relying on real data. Developers can create a virtual environment which allows players to interact directly with the AI. The machine can then learn from the game's data, making better decisions.
FAQ
Which countries lead the AI market and why?
China has more than $2B in annual revenue for Artificial Intelligence in 2018, and is leading the market. China's AI industry is led in part by Baidu, Tencent Holdings Ltd. and Tencent Holdings Ltd. as well as Huawei Technologies Co. Ltd. and Xiaomi Technology Inc.
China's government is heavily investing in the development of AI. The Chinese government has created several research centers devoted to improving AI capabilities. These centers include the National Laboratory of Pattern Recognition and State Key Lab of Virtual Reality Technology and Systems.
Some of the largest companies in China include Baidu, Tencent and Tencent. All of these companies are currently working to develop their own AI solutions.
India is another country that is making significant progress in the development of AI and related technologies. The government of India is currently focusing on the development of an AI ecosystem.
Which industries use AI the most?
The automotive sector is among the first to adopt AI. BMW AG uses AI as a diagnostic tool for car problems; Ford Motor Company uses AI when developing self-driving cars; General Motors uses AI with its autonomous vehicle fleet.
Other AI industries include banking, insurance, healthcare, retail, manufacturing, telecommunications, transportation, and utilities.
How does AI work
An algorithm is a set or instructions that tells the computer how to solve a particular problem. A sequence of steps can be used to express an algorithm. Each step is assigned a condition which determines when it should be executed. A computer executes each instruction sequentially until all conditions are met. This repeats until the final outcome is reached.
Let's suppose, for example that you want to find the square roots of 5. You could write down every single number between 1 and 10, calculate the square root for each one, and then take the average. This is not practical so you can instead write the following formula:
sqrt(x) x^0.5
This means that you need to square your input, divide it with 2, and multiply it by 0.5.
Computers follow the same principles. It takes the input and divides it. Then, it multiplies that number by 0.5. Finally, it outputs its answer.
What is the most recent AI invention
The latest AI invention is called "Deep Learning." Deep learning is an artificial Intelligence technique that makes use of neural networks (a form of machine learning) in order to perform tasks such speech recognition, image recognition, and natural language process. Google invented it in 2012.
Google recently used deep learning to create an algorithm that can write its code. This was achieved by a neural network called Google Brain, which was trained using large amounts of data obtained from YouTube videos.
This allowed the system to learn how to write programs for itself.
IBM announced in 2015 they had created a computer program that could create music. The neural networks also play a role in music creation. These are sometimes called NNFM or neural networks for music.
What does the future look like for AI?
The future of artificial intelligence (AI) lies not in building machines that are smarter than us but rather in creating systems that learn from experience and improve themselves over time.
We need machines that can learn.
This would allow for the development of algorithms that can teach one another by example.
You should also think about the possibility of creating your own learning algorithms.
The most important thing here is ensuring they're flexible enough to adapt to any situation.
Who is the inventor of AI?
Alan Turing
Turing was born 1912. His father was clergyman and his mom was a nurse. He excelled in mathematics at school but was depressed when he was rejected by Cambridge University. He learned chess after being rejected by Cambridge University. He won numerous tournaments. He returned to Britain in 1945 and worked at Bletchley Park's secret code-breaking centre Bletchley Park. Here he discovered German codes.
He died in 1954.
John McCarthy
McCarthy was born in 1928. He studied maths at Princeton University before joining MIT. There he developed the LISP programming language. In 1957, he had established the foundations of modern AI.
He died in 2011.
What are some examples of AI applications?
AI is used in many areas, including finance, healthcare, manufacturing, transportation, energy, education, government, law enforcement, and defense. Here are just a few examples:
-
Finance - AI can already detect fraud in banks. AI can identify suspicious activity by scanning millions of transactions daily.
-
Healthcare – AI is used for diagnosing diseases, spotting cancerous cells, as well as recommending treatments.
-
Manufacturing - AI can be used in factories to increase efficiency and lower costs.
-
Transportation - Self driving cars have been successfully tested in California. They are being tested across the globe.
-
Utilities are using AI to monitor power consumption patterns.
-
Education - AI is being used in education. Students can use their smartphones to interact with robots.
-
Government - AI can be used within government to track terrorists, criminals, or missing people.
-
Law Enforcement – AI is being utilized as part of police investigation. Search databases that contain thousands of hours worth of CCTV footage can be searched by detectives.
-
Defense - AI is being used both offensively and defensively. An AI system can be used to hack into enemy systems. Protect military bases from cyber attacks with AI.
Statistics
- While all of it is still what seems like a far way off, the future of this technology presents a Catch-22, able to solve the world's problems and likely to power all the A.I. systems on earth, but also incredibly dangerous in the wrong hands. (forbes.com)
- In the first half of 2017, the company discovered and banned 300,000 terrorist-linked accounts, 95 percent of which were found by non-human, artificially intelligent machines. (builtin.com)
- Additionally, keeping in mind the current crisis, the AI is designed in a manner where it reduces the carbon footprint by 20-40%. (analyticsinsight.net)
- According to the company's website, more than 800 financial firms use AlphaSense, including some Fortune 500 corporations. (builtin.com)
- The company's AI team trained an image recognition model to 85 percent accuracy using billions of public Instagram photos tagged with hashtags. (builtin.com)
External Links
How To
How to set up Amazon Echo Dot
Amazon Echo Dot can be used to control smart home devices, such as lights and fans. To begin listening to music, news or sports scores, say "Alexa". You can ask questions, make phone calls, send texts, add calendar events, play video games, read the news and get driving directions. You can also order food from nearby restaurants. Bluetooth speakers or headphones can be used with it (sold separately), so music can be played throughout the house.
You can connect your Alexa-enabled device to your TV via an HDMI cable or wireless adapter. An Echo Dot can be used with multiple TVs with one wireless adapter. You can pair multiple Echos together, so they can work together even though they're not physically in the same room.
Follow these steps to set up your Echo Dot
-
Your Echo Dot should be turned off
-
The Echo Dot's Ethernet port allows you to connect it to your Wi Fi router. Make sure that the power switch is off.
-
Open the Alexa app on your phone or tablet.
-
Select Echo Dot in the list.
-
Select Add New.
-
Select Echo Dot (from the drop-down) from the list.
-
Follow the screen instructions.
-
When asked, enter the name that you would like to be associated with your Echo Dot.
-
Tap Allow Access.
-
Wait until Echo Dot has connected successfully to your Wi Fi.
-
Repeat this process for all Echo Dots you plan to use.
-
Enjoy hands-free convenience